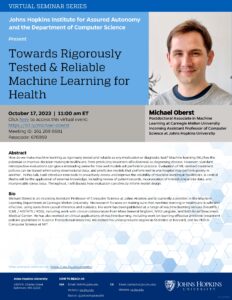
Abstract: How do we make machine learning as rigorously tested and reliable as any medication or diagnostic test? Machine learning (ML) has the potential to improve decision-making in healthcare, from predicting treatment effectiveness to diagnosing disease. However, standard retrospective evaluations can give a misleading sense for how well models will perform in practice. Evaluation of ML-derived treatment policies can be biased when using observational data, and predictive models that perform well in one hospital may perform poorly in another. In this talk, I will introduce new tools to proactively assess and improve the reliability of machine learning in healthcare. A central theme will be the application of external knowledge, including review of patient records, incorporation of limited clinical trial data, and interpretable stress tests. Throughout, I will discuss how evaluation can directly inform model design.
Bio: Michael Oberst is an incoming Assistant Professor of Computer Science at Johns Hopkins and is currently a postdoc in the Machine Learning Department at Carnegie Mellon University. His research focuses on making sure that machine learning in healthcare is safe and effective, using tools from causal inference and statistics. His work has been published at a range of machine learning venues (NeurIPS / ICML / AISTATS / KDD), including work with clinical collaborators from Mass General Brigham, NYU Langone, and Beth Israel Deaconess Medical Center. He has also worked on clinical applications of machine learning, including work on learning effective antibiotic treatment policies (published in Science Translational Medicine). He earned his undergraduate degree in Statistics at Harvard, and his PhD in Computer Science at MIT.
Zoom: https://jhuapl.zoomgov.com/j/1612696681?pwd=L2l4cXZaYzU5cS96VmVwZ3R2bWJPZz09
Meeting ID: 161 269 6681
Passcode: 676959