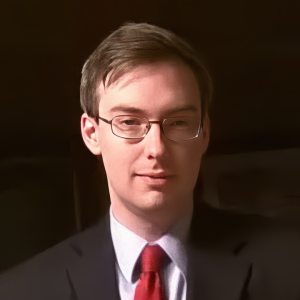
Title: Bridging the Human-Machine Information Gap for AI Fighter Pilots
Abstract: We discuss the research and development of an AI copilot that predicts human pilot decision making and offers time-critical recommendations to enable machine-precision decisions at machine speeds. The latest in a long line of innovation in AI for air combat at the Johns Hopkins University Applied Physics Laboratory (APL), this AI copilot is an autonomous agent that can function simultaneously as a situationally-aware peer, a cognitive support assistant, and a highly-performant fighter jet pilot. In addition, the AI agent can also serve as a loyal wingman, flying one or more uncrewed autonomous vehicles that can respond dynamically in real time to human commands. Achieving this behavior required innovating new forms of machine learning, including recurrent variational autoencoders for imitation learning, graph neural networks for coordinated behavioral prediction, and novel transformer architectures for dynamic multi-tasking and zero-shot generalization. These AI copilot and wingmen agents are integrated into a high-fidelity virtual reality cockpit that enables interactive demonstrations and experimentation of human-machine teaming scenarios.
Bio: Dr. John Winder is a computer scientist and supervisor of the Advanced AI Algorithms section at the Johns Hopkins University Applied Physics Laboratory (APL). At APL, he leads a team researching AI and machine learning for complex, real-world systems. His recent work encompasses multi-agent reinforcement learning (RL) for cooperative-competitive environments, multi-task knowledge transfer, and human-machine teaming. John joined APL in 2020 after receiving his PhD from UMBC, where his doctoral work focused on hierarchical RL for abstract decision making and concept formation to increase generalization and create more adaptable agent-based AI.
Zoom: https://jhuapl.zoomgov.com/j/1602659447?pwd=wuDPqeecjPYRwvbUbwUAKPIpT3HmZk.1
Meeting ID: 160 265 9447
Passcode: 241294