Alan Yuille, Johns Hopkins Whiting School of Engineering
Yinzhi Cao, Johns Hopkins Whiting School of Engineering
Philippe Burlina, Johns Hopkins Applied Physics Laboratory
Deep learning (DL) systems can be vulnerable to malicious attacks, as demonstrated by recent successes in adversarial machine learning (AML). This vulnerability presents one of the main obstacles to deploying trustworthy AI in autonomous systems. This research is broken into three complementary activities: (1) novel methods for generating physical AML attacks, (2) novel state-of-the-art algorithms for defending against these attacks, and (3) a testing and evaluation framework for experimentation under controlled and real-world settings.
The primary focus of this research is to increase the resilience of DL systems against both physical patch-based attacks, where a compact pattern is optimized and placed to fool a DL system, and occlusion-based attacks, where portions of the scene are strategically obscured to attack a system behavior. This research will use a black-box method for generating attacks using transformations to identify system vulnerabilities and deficiencies, which in turn will be leveraged to improve system robustness.
This work is poised to have far-ranging impact in many domains—transportation, medical, and smart cities/campuses among them—by increasing the reliability of autonomous systems that use DL for sensing and decision-making.
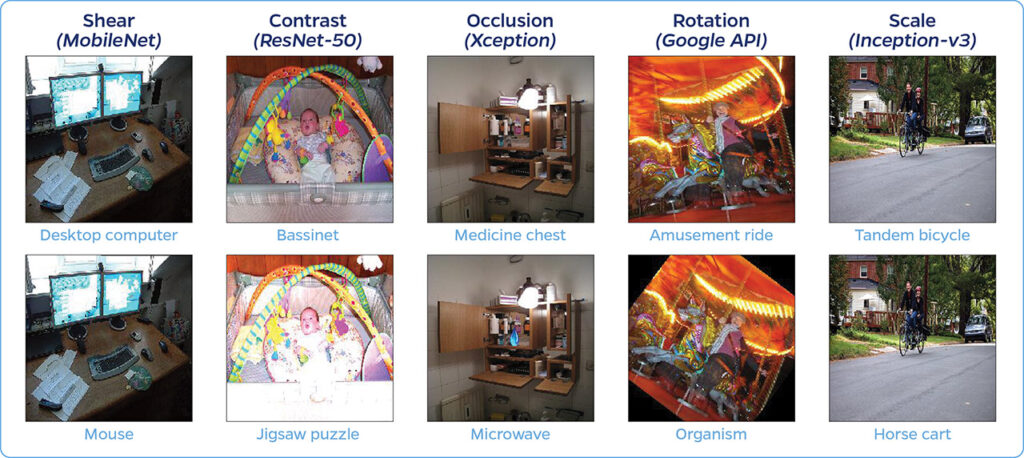
Figure 1: Upper row shows the original inputs. Lower row shows the violations generated by different transformations for different computer vision systems.